Alon Kipnis
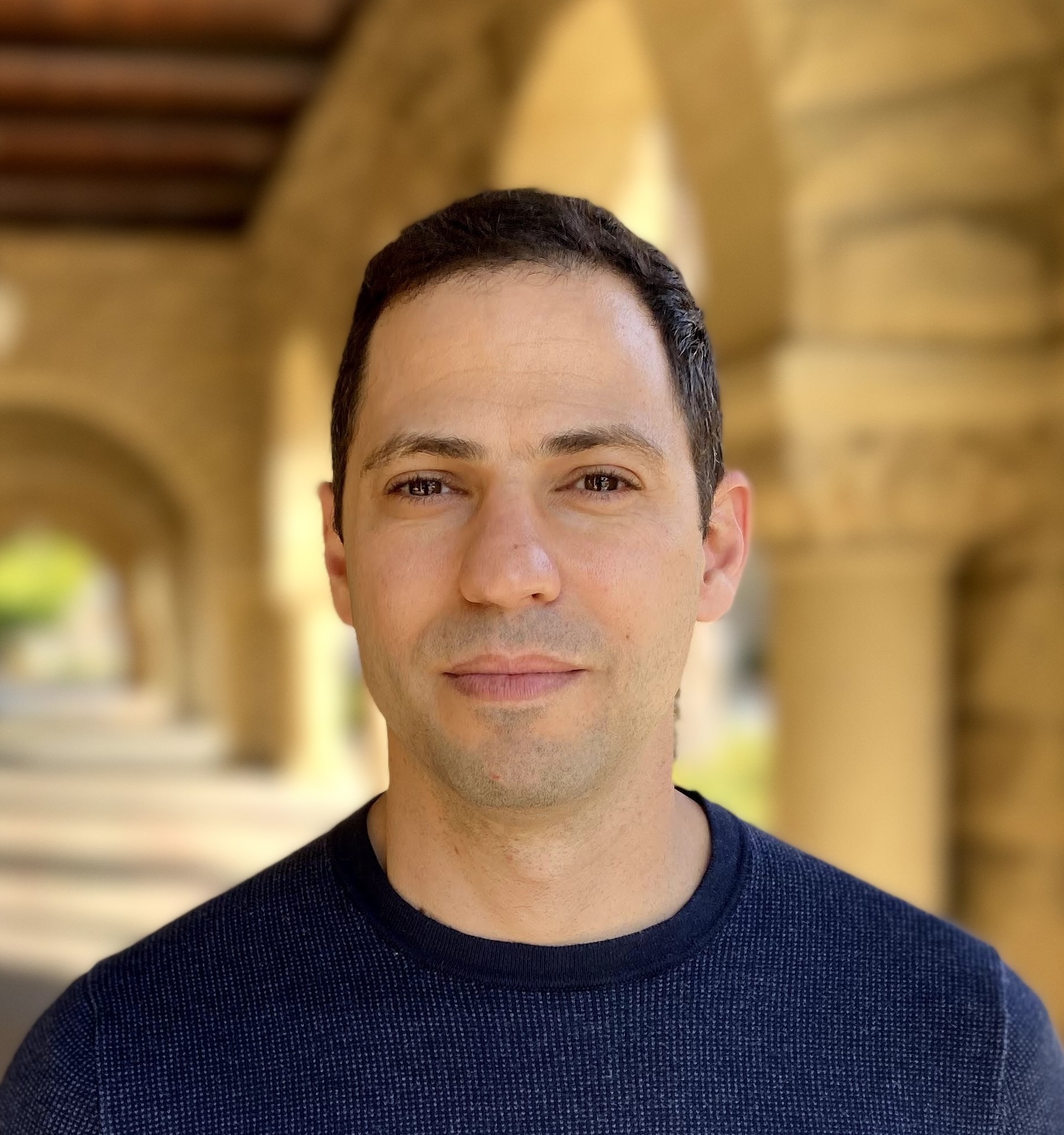
About
I'm a Senior Lecturer (Assistant Professor) in the School of Computer Science at Reichman University. I received my PhD in Electrical Engineering from Stanford University in 2017, advised by Andrea Goldsmith. From 2017 to 2021, I was a postdoctoral scholar and lecturer in the Department of Statistics at Stanford, advised by David Donoho.
My research lies at the intersection of mathematical statistics, information theory, and signal processing. I focus on understanding and developing methods for analyzing high-dimensional, sparse, and noisy data — including time-series and text. My work blends rigorous mathematical theory with ambitious computational experiments and data analysis.
I'm always on the lookout for motivated graduate students with a strong mathematical background and a passion for data science. If that sounds like you, take a look at my current list of open projects.
Contact
Office Hours (Spring 2024/2025)
Tuesdays, 14:00-15:00
Address
C.121b
Efi Arazi School of Computer Science
Reichman University (formerly IDC)
Herzliya, Israel
alon.kipnis@runi.ac.il
kipnisal@alumni.stanford.edu
Publications
Journal publications under review
Tingnan Gong, Alon Kipnis, and Yao Xie. (2025). Higher-criticism for sparse multi-stream change-point detection
Alon Kipnis. (2024). The minimax risk in uniformity testing of poisson data under missing ball alternatives
Ben Galili, Alon Kipnis, and Zohar Yakhini. (2023). Detecting sparse and weak deviations of non-proportional hazard in survival analysis
Journal Publications
Shira Faigenbaum-Golovin, Alon Kipnis, Axel Bühler, Eli Piasetzky, Thomas Römer, and Israel Finkelstein. (2025) Critical biblical studies via word frequency analysis: unveiling text authorship. PLOS One
Alon Kipnis. (2025). Unification of rare/weak detection models using moderate deviations analysis and log-chisquared P-values. Statistica Sinica
Idan Kashtan and Alon Kipnis (2024). An information-theoretic approach for detecting edits in AI-generated text. Harvard Data Science Review
David L. Donoho and Alon Kipnis. (2024). The impossibility region for detecting sparse mixtures using the higher criticism. Annals of Applied Probability
Alon Kipnis and John C. Duchi. (2022). Mean estimation from one-bit measurements. IEEE Transactions on Information Theory
David L. Donoho and Alon Kipnis. (2022). Higher Criticism to compare two large frequency tables, with sensitivity to possible rare and weak differences. Annals of Statistics
Alon Kipnis. (2022). Higher criticism for discriminating word-frequency tables and testing authorship. Annals of Applied Statistics
Alon Kipnis and Galen Reeves. (2021). Gaussian approximation of quantization error for estimation from compressed data. IEEE Transactions on Information Theory
Alon Kipnis, Stefano Rini, and Andrea J. Goldsmith. (2021). The rate-distortion risk in estimation from compressed data. IEEE Transactions on Information Theory
Stefano Rini, Alon Kipnis, Ruiyang Song, and Andrea J. Goldsmith. (2019). The compress-and-estimate coding scheme for Gaussian sources. IEEE Transactions on Wireless Communications
Alon Kipnis, Andrea J. Goldsmith and Yonina C. Eldar. (2018). The distortion rate function of sampled Wiener Processes. IEEE Transactions on Information Theory
Alon Kipnis, Andrea J. Goldsmith, and Yonina, C. Eldar. (2018). Fundamental distortion limits of analog to digital compression. IEEE Transactions on Information Theory
Alon Kipnis, Yonina C. Eldar, and Andrea J. Goldsmith. (2018). Analog-to-digital compression: a new paradigm for converting signals to bits. IEEE Signal Processing Magazine
Alon Kipnis, Andrea J. Goldsmith and Yonina, C. Eldar. (2017). function of cyclostationary Gaussian processes/. IEEE Transactions on Information Theory
Alon Kipnis, Andrea J. Goldsmith, Yonina, C. Eldar and Tsachy Weissman. (2016). Distortion rate function of sub-Nyquist sampled Gaussian sources. IEEE Transactions on Information Theory
Daniel Alpay and Alon Kipnis. (2015). Wiener chaos approach to optimal prediction. Numerical Functional Analysis and Optimization
Daniel Alpay and Alon Kipnis. (2013). A generalized white noise space approach to stochastic integration for a class of Gaussian stationary increment processes. Opuscula Mathematica
Book Chapters
Alon Kipnis, Yonina C. Eldar and Andrea J. Goldsmith. (2021). An information-theoretic approach to analog-to-digital compression
Refereed Conference Publications
Alexander Tsvetkov and Alon Kipnis. Information parity: Measuring and predicting the multilingual capabilities of language models. Findings of the Association for Computational Linguistics (EMNLP) 2024
Tingnan Gong, Alon Kipnis and Yao Xie. Higher-criticism for sparse multi-sensor change-point detection. 60th Annual Allerton Conference on Communication, Control, and Computing 2024
Alexander Tsvetkov and Alon Kipnis. multilingual compression parity: How efficiently large language models represent information across languages? ICML 2024 Workshop on Theoretical Foundations of Foundation Models
Dana Levin and Alon Kipnis. The likelihood gain of a language model as a metric for text summarization. IEEE International Symposium on Information Theory 2024.
Alon Kipnis. The minimax risk in testing the histogram of discrete distributions for uniformity under missing ball alternatives. 59th Annual Allerton Conference on Communication, Control, and Computing 2023
Alexander Tsvetkov and Alon Kipnis. EntropyRank: Unsupervised keyphrase extraction via side-information optimization for language model-based text compression ICML 2023 Workshop on Neural Compression: From Information Theory to Applications
Alon Kipnis. Rare and weak detection models under moderate deviations analysis and log-chisquared p-values. IEEE International Symposium on Information Theory 2022
Alon Kipnis and David L. Donoho. Two-sample testing of word-frequency tables under rare/weak Perturbations. IEEE International Symposium on Information Theory 2021
Alon Kipnis. Higher criticism as an unsupervised authorship discriminator. Conference and Labs of the Evaluation Forum 2020
Alon Kipnis and Galen Reeves. Gaussian approximation of quantization error for estimation from compressed data. IEEE International Symposium on Information Theory 2019
Alon Kipnis and Galen Reeves. Single-letter formulas for quantized compressed sensing with Gaussian codebooks. IEEE International Symposium on Information Theory 2019
Alon Kipnis, Galen Reeves, and Yonina C. Eldar. Single-letter formulas for quantized compressed sensing with Gaussian codebooks. IEEE International Symposium on Information Theory 2018
Georgia Murray, Alon Kipnis, and Andrea J. Goldsmith. Lossy compression of decimated Gaussian random walks. 52nd Annual Conference on Information Sciences and Systems 2018
Alon Kipnis and John C. Duchi. Mean estimation from adaptive one-bit measurements. 55th Annual Allerton Conference on Communication, Control, and Computing 2017
Alon Kipnis, Galen Reeves, Yonina C. Eldar and Andrea J. Goldsmith. Compressed sensing under optimal quantization. IEEE International Symposium on Information Theory 2017
Alon Kipnis, Stefano Rini, and Andrea J. Goldsmith. Coding theorems for the compress and estimate source coding problem. IEEE International Symposium on Information Theory 2017
Ruyang Song, Stefano Rini, Alon Kipnis, and Andrea J. Goldsmith. Optimal rate-allocation in multiterminal compress-and-estimate source coding. IEEE Information Theory Workshop 2016
Alon Kipnis, Andrea J. Goldsmith, and Yonina C. Eldar, Information rates of sampled Wiener processes. IEEE International Symposium on Information Theory 2016
Alon Kipnis, Stefano Rini, and Andrea J. Goldsmith. emph{Multiterminal compress-and-estimate source coding}. IEEE International Symposium on Information Theory 2016
Milind Rao, Alon Kipnis, Tara Javidi, Yonina C. Eldar and Andrea J. Goldsmith. System identification from partial samples: non-asymptotic analysis. IEEE 55th Conference on Decision and Control 2016
Alon Kipnis, Andrea J. Goldsmith and Yonina C. Eldar. Optimal trade-off between sampling rate and quantization precision in A/D conversion. 53rd Annual Allerton Conference on Communication, Control, and Computing 2015
Alon Kipnis, Stefano Rini, and Andrea J. Goldsmith. The indirect rate-distortion function of a binary i.i.d source. IEEE Information Theory Workshop 2015
Mainak Chowdhury, Alon Kipnis, and Andrea J. Goldsmith. Reliable uncoded communication in the quantized SIMO MAC. IEEE International Symposium on Information Theory 2015
Alon Kipnis, Andreea J. Goldsmith, and Yonina C. Eldar. Optimal trade-off between sampling rate and quantization precision in Sigma-Delta A/D conversion. International Conference on Sampling Theory and Applications 2015
Alon Kipnis, Andrea J. Goldsmith, and Yonina C. Eldar. Sub-Nyquist sampling achieves optimal rate-distortion. IEEE Information Theory Workshop 2015
Alon Kipnis, Andrea J. Goldsmith, and Yonina C. Eldar. Rate-distortion function under sub-Nyquist nonuniform sampling. 52st Annual Allerton Conference on Communication, Control, and Computing 2014
Alon Kipnis, Andrea J. Goldsmith, and Yonina C. Eldar. Rate-distortion function of Gaussian Cyclostationary processes. IEEE International Symposium on Information Theory 2014
Alon Kipnis, Andrea J. Goldsmith, Tsachy Weissman and Yonina C. Eldar. Rate-distortion function of sub-Nyquist sampled Gaussian processes corrupted by noise. 51st Annual Allerton Conference on Communication, Control, and Computing 2013
Dissertations
Alon Kipnis. (2017). Fundamental performance limits of analog-to-digital compression
Alon Kipnis. (2012). Generalized white noise space anlysis and stochastic integration with respect to a class of Gaussian stationary increment processes
Patents
See this page, or search it yourself at SenseIP https://www.senseip.ai/.
Teaching
Office hours: Tuesdays. 14:00-15:00 Location: C.121b
Current (Spring 2024/5)
Machine Learning from Data (CS 3141)
Seminar in Large language Models and Information Theory (CS 3968)
Students’ presentations at https://github.com/alonkipnis/ITnLM
Past
Fall 2024/5:
Advanced statistical analysis and model-based learning (formerly ‘Advanced Statistics for Data Science’) (CS 3676)
Spring 2023/4:
Machine Learning from Data (CS 3141)
Seminar in Large language Models and Information Theory (CS 3968)
Fall 2023/4:
Introduction to Information Theory (CS 3798)
Spring 2022/3:
Machine Learning from Data (CS 3141)
Advanced statistics for data science (CS 3676)
Fall 2022/3:
Information-theoretical analysis of neural language models (CS 3890)
Spring 2021/2:
Advanced statistics for data science (CS 3676)
Spring 2021/2: (Stanford STATS 285) Massive computational experiments, painlessly
with David Donoho and Masha Lofti
Fall 2020/1: (Stanford STATS 207) Introduction to time-series analysis (Fall 2020-2021)
Lecture 1 | course outline, examples of time series data, models for time series data |
Lecture 2 | sample autocorrelation and basic theoretical constructs |
Lecture 3 | time series regression |
Lecture 4 | trend models and Data Wrangling |
Lecture 5-6 | ARMA/ARIMA Modeling I |
Lecture 7 | ARMA/ARIMA Modeling II |
Lecture 8 | ARIMA/SARIMA |
Lecture 9 | regression with autocorrelated errors and lagged regression |
Lecture 10 | efficient markets hypothesis and GARCH (guest lecture by David Donoho) |
Lecture 11 | spectral Analysis I |
Lecture 12 | spectral Analysis II |
Lecture 13 | spectral regression and principal components |
Lecture 14 | state-space modeling and the Kalman Filter |
Lecture 15 | estimation of state-space models |
Lecture 16 | dynamic linear models with switching |
Lecture 17 | stochastic volatility, Bayesian analysis of state-space models |
Lecture 18 | bootstrap reality check and technical trading rules (guest lecture by David Donoho) |
Lecture 19 | prophet (guest lecture by Sean Taylor) |
Lecture 20 | high-dimensional data, DeepAR, VEST |
Available Research Projects
Goodness-of-fit and homogeneity testing with sparse data
Information-theoretic limitations of large language models
Multi-channel time-series prediction
Test clustering, retreival, and topic modeling
Survival analysis
Change-point detection